Data Science & Climate
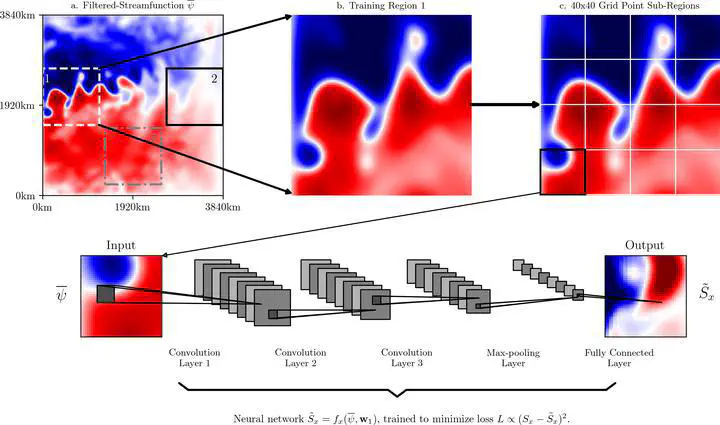
We work on data-driven methods to undertand and predict climate. The tools we use range from probabilistic models informed by data to machine learning algorithms (e.g., neural networks).
Some of our data-driven work include:
– Simple statistical models for prediction of seasonal to decadal sea surface temperatures in the Atlantic (e.g., Zanna 2012, Huddart et al. 2016) and Pacific (e.g., Dias et al. 2018), and sea level (e.g., Fraser et al. 2019).
– Data-driven scale- and flow-aware determinitic and stochastic parametrizations of ocean turbulence (e.g., Porta Mana & Zanna 2014, Zanna et al. 2017, David et al. 2017).
– Data inference using machine learning (e.g., Bolton and Zanna, 2019).
– Representation of climate processes with Neural Net and Equation-Discovery (e.g., Bolton and Zanna, 2019, Bolton and Zanna, 2019).
We are actively using machine learning and more generally data-driven tools to address some of our favorite problems related to parameterization of turbulent processes, interannual to decadal prediction, and forecasting of extreme events.