Data-driven dimensionality reduction and causal inference for spatiotemporal climate fields
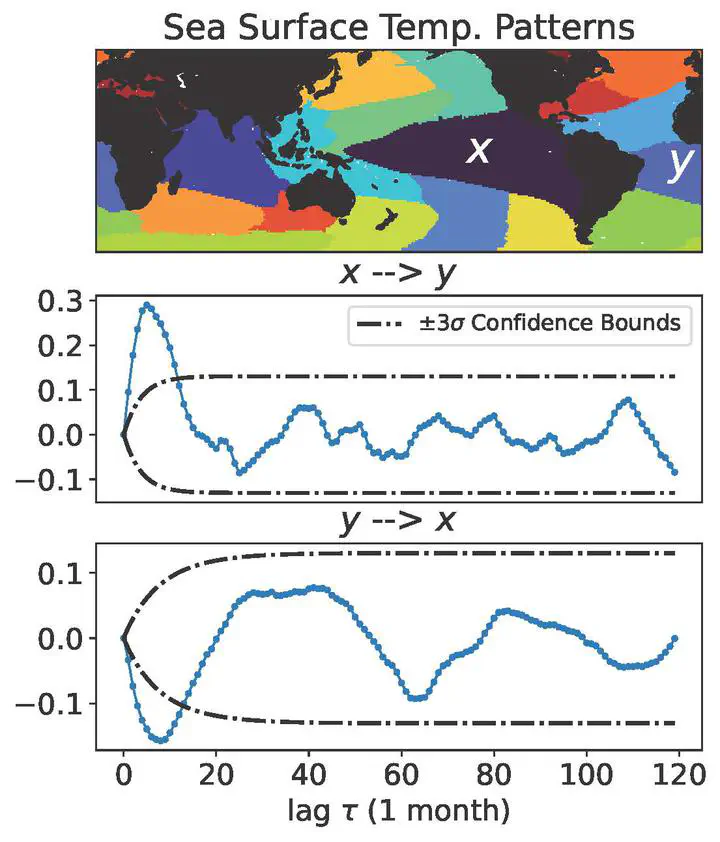
One of the main challenges in statistical inference is to infer causal relations rather than simple correlations. In this paper, Fabrizio Falasca, Pavel Perezhogin, and Laure Zanna, propose a framework, based on linear response theory, to investigate and diagnose causal mechanisms in the climate system directly from data. The method is physically-based and scales to high-dimensional systems. Possible future applications include the response of the climate system to external perturbations and evaluate climate models outputs.