Benchmarking of machine learning ocean subgrid parameterizations in an idealized model
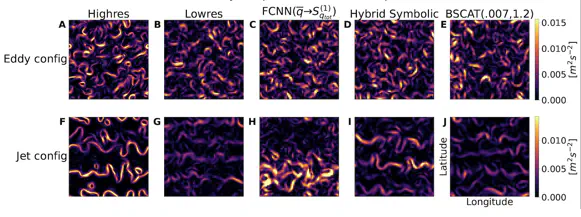
New preprint by Ross et al.
In this work, led by Andrew Ross, with Ziwei Li, Pavel Perezhogin, Carlos Fernandez-Granda and Laure Zanna, we provide a framework for systematically benchmarking the offline and online performance of physical and ML-based subgrid parameterizations. We find that the choice of filtering operator is critical for performance. To help with interpretability, we also propose a novel equation-discovery approach combining linear regression and genetic programming which generalizes better than physical and neural network parameterizations.
See code and notebooks here: https://github.com/m2lines/pyqg_parameterization_benchmarks