M²LInES - New Collaboration on Machine Learning for Climate Modeling support by Schmidt Futures
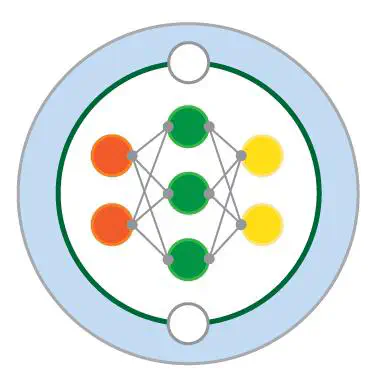
Experts in Machine learning, Climate Physics, and Modeling team up to deepen our understanding of climate
Read our press release.
We are excited to announce the launch of our new international project M²LInES – Multiscale Machine Learning In coupled Earth System Modeling – aimed at deepening our understanding of climate processes, and improving climate projections using scientific machine learning. Our team includes scientists from New York University, Princeton, GFDL, Columbia, LDEO, NCAR, MIT, CNRS-IGE, and CNRS-IPSL.
The overall goal of the project is to improve climate projections and reduce climate model biases, especially at the air-sea interface, using machine learning (ML). We will rely on data from a range of high-resolution (idealized and global) simulations and data assimilation products to deepen our understanding and improve the representation of subgrid physics in the ocean, sea-ice and atmosphere components of existing IPCC-class climate models. In addition, we will work on overcoming challenges related to ML for climate modeling including sampling efficiency, generalization, interpretability and uncertainty quantification.
We are looking for 1 project manager and 12 postdocs right now at several institutions, please visit https://m2lines.github.io/jobs/ for more info about the different positions available, and how to apply. This is a highly collaborative project, and the researchers are expected to interact with different groups.